Density Functional Theory with Machine Learned Functional
R. Nagai, R. Akashi, and O. Sugino
Modern electronic structure theory of materials is most frequently based on the Kohn-Sham density functional theory (KS-DFT), where the accuracy depends on the quality of the density-to-energy mapping called exchange-correlation (xc) functional. The mapping has extremely large degrees of freedom that are too complex to determine manually. In this context, we have proposed a machine learning approach [1]. We have prepared the atomization energy (Ea) and electron density n(r) of reference molecules using a quantum chemical simulation and let neural network (NN) learn the data. In the learning, the energy Ea and the density n(r) are related via a functional of the density called the xc potential εxc[n](r), and the parameters characterizing the functional are optimized to best reproduce the input data. The mapping thus established depends on the form of the functional, such as the point-to-point mapping called local density approximation (LDA) and the mapping reinforced using the density gradient called generalized gradient approximation (GGA) or meta-GGA. Quality of the functionals was tested for a ten dozen of unreferenced molecules and was found superior to existing ones for each form, suggesting possibility to systematically develop accurate functionals.
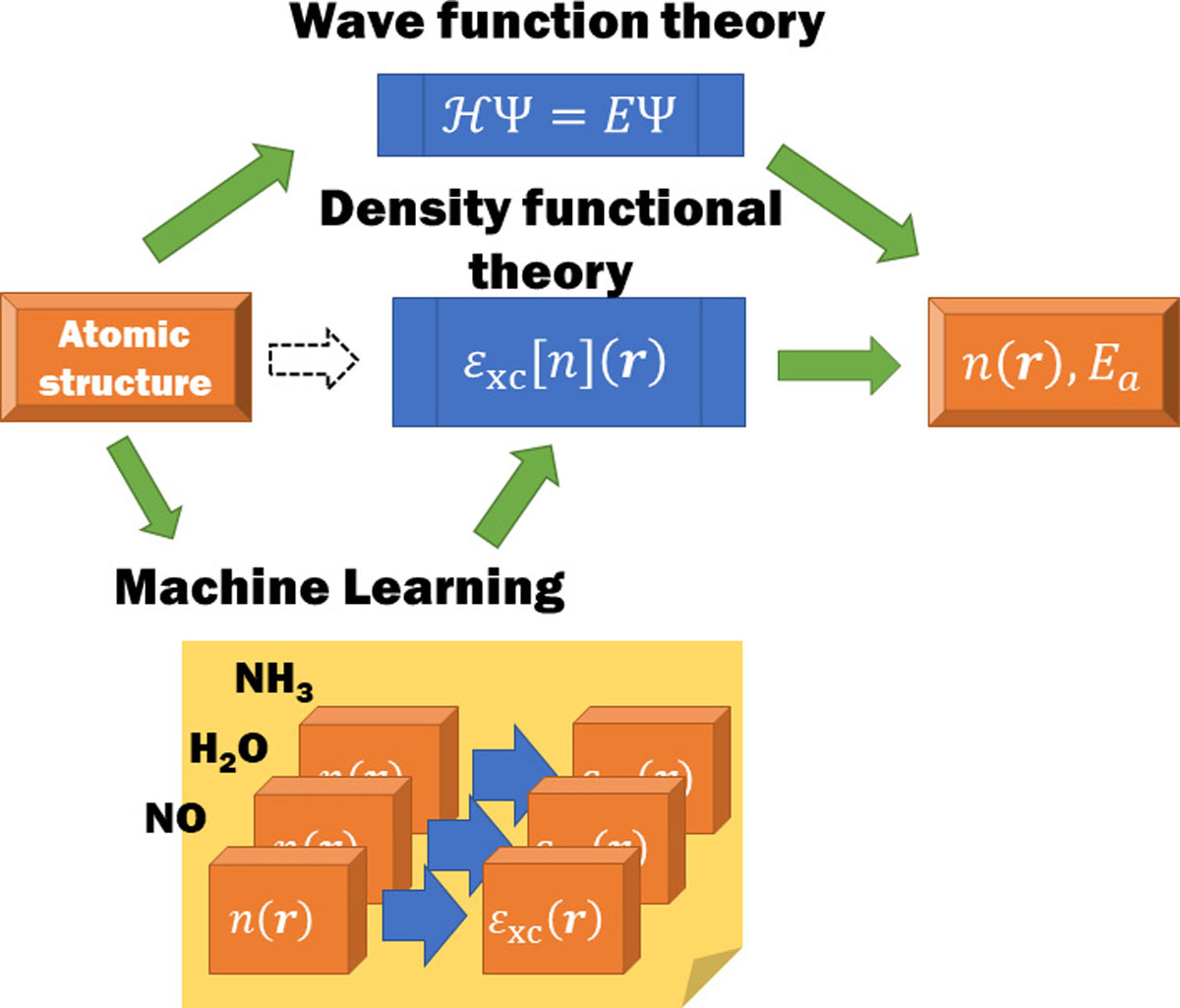
Fig. 1. Machine learning scheme for developing exchange correlation (xc) functional. Referring to electron density n(r) and atomization energy Ea of a few molecules, the xc energy was related to the density as a functional εxc[n](r). With this machine learned functional, n(r) and Ea of unrefernced materials were found to be predicted more accurately than with conventional functionals.
In our study, we have used three molecules, H2O, NH3, and NO, that contain different bonding property and spin polarization, as the reference. The NN successfully determined the parameters without overtraining owing large number of data contained in the density, which amounts to 100,000. We further tried to include nonlocality into the functional beyond the gradient approximations by considering the electron density averaged in the vicinity of an electron. The resulting functional form, called near region approximation (NRA), improves the accuracy equivalent to that achieved using the nonlocal exchange, allowing thereby significantly to reduce the computational complexity.
In view of the ability of artificial intelligence to recognize a picture, it is likely to be possible to learn the chemical bonds without explicitly indicating a descriptor. Such advance in the method will enable a researcher to develop a high-quality functional dedicated to the target materials of interest with an effect of speed-up of material design. Impact of systematic functional development is thus expected to be large in the computational materials science.
References
- [1] R. Nagai, R. Akashi, and O. Sugino, npj Compt. Mater. 6, 43 (2020).